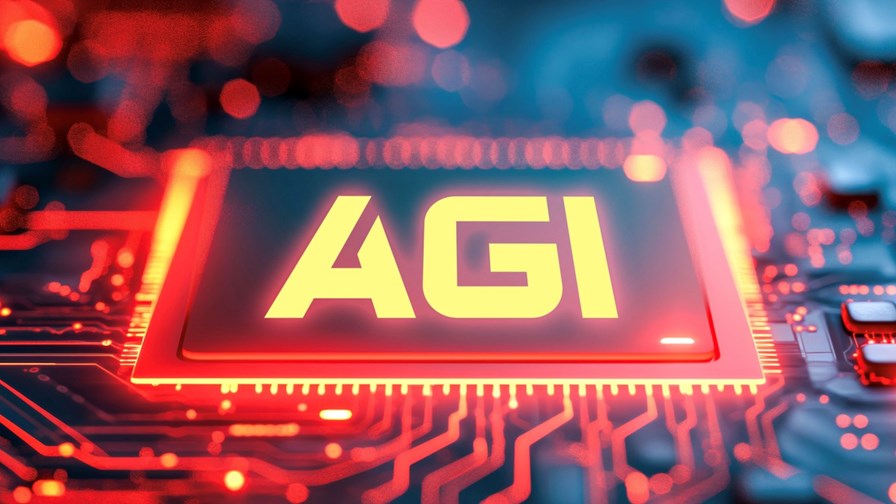
- Multimodal AI can do more than today’s artificial narrow intelligence but…
- …it is also based on large language model training and, therefore, it’s potential is inherently limited
- It is not possible to achieve artificial general intelligence (AGI) without a completely new approach
- But much is invested in the current model and the production of specially designed GPU semiconductors that make it work
The pace of artificial intelligence (AI) development is unrelenting and is now increasingly focused on processing much more than just written prompts – but might current developments, underpinned by billions of dollars of investment, be taking the AI sector down a cul-de-sac?
Today, many people’s daily online interactions, either for work or for leisure, are moderated by generative AI (GenAI) systems. Like it or not, we are all now, perforce, accustomed to interaction with text-based artificial intelligence chatbots, which are globally ubiquitous. And text is just the start: As GenAI continues to develop, new ‘multimodal’ models are being introduced that can process images, video, audio and speech as well as text.
Multimodal AI trains using multiple types of data input and a wide range of different types of numerical datasets: Earlier versions of GenAI could not perform such complex operations as they were trained on a single type or source of data for a particular purpose, such as for the financial sector. In multimodal AI the data types are used together to enable the interpretation of context, the determination of better informed outcomes and establishment of more sophisticated, detailed and accurate content.
Nonetheless, as is the case with the first models of generative AI, multimodal AI is, in turn, also built and reliant on large language model (LLM) software to scrape and appropriate data (often without permission) from a myriad of sources, much of which is supposed to be under the protection of copyright laws. New algorithms regulate how the enlarged datasets help build the several neural networks dedicated to a particular type of data, be that speech, video, audio or text. These are brought together in a ‘fusion module’ that combines and processes separate data streams, interprets them and transmits output responses. Those outputs become part of a user feedback loop that is used further to improve and extend the AI.
The ultimate goal is to render multimodal AI capable of, at least, mimicking human perception, and beguiling users into thinking AI is just like us. But it isn’t. The similarity is barely skin-deep. The AI we know today is actually best described as artificial narrow intelligence (ANI) and ANI is dedicated to one specific task, such as facial recognition technology, and in devices like Siri and Amazon’s Alexa, as well as Tesla’s self-driving cars. The data they use cannot be transferred and applied in other domains for which they have not been, and cannot, be trained.
The race to build artificial general intelligence
Hence the race to develop artificial general intelligence (AGI ) that will be able to operate in a wide range of environments. It’s a race that is happening in a miasma of hyperbole and in the absence of any generally agreed overarching definition of what would constitute AGI.
Based, as it is, on current unimodal AI models, it seems doomed to failure. Nonetheless, in September, Sam Altman, the CEO at OpenAI, which developed the application (ChatGPT) that arguably took GenAI to the mass market, opined that it might be a reality within “a few thousand days”. Meanwhile, Elon Musk reckons it will be with us by 2026. (Isn’t he supposed to be resident on Mars by then? Perhaps he knows something we don’t…)
On 11 October, the Wall Street Journal published an interview with Yan LeCun, a renowned AI pioneer, professor of AI at New York University, oft-quoted AI guru and head of research at Meta. He told the newspaper that doom-mongering warnings that AI could soon become an existential threat to the future of humanity are “complete BS”. He admits that while the AI models of today are “useful tools” he insists that they are not, “in any meaningful way, intelligent”, and are nowhere near the point of exceeding human capabilities. Indeed, they may never do so.
In a posting on X earlier this year, Yan LeCun wrote, “It seems to me that before urgently figuring out how to control AI systems much smarter than us, we need to have the beginning of a hint of a design for a system smarter than a house cat.”
As he points out, domestic moggies do have a mental model of the world that they inhabit, they do have a long-term and short-term memory, some limited reasoning ability and, to a minimal extent, they can plan. However, limited though a cat’s intelligence is, none of the attributes they do have can be ascribed in any way to any of today’s AI models.
LeCun adds that it simply won’t be possible to get to AGI from where we are today because the models we are using are not designed to do the job. Large language models do little more than scrape and modify masses of data and many AI companies have adopted a strategy of adding more and more specially designed GPU semiconductors to their datacentre systems in the hope that, eventually, they will somehow produce an intelligence greater than in humans. The professor says it won’t happen because it can’t happen. The reality is that LLMs will have only a minor role in the evolution of AGI because of the limitations of their models.
He says that all artificial narrow intelligence does is predict the next word in a text and that we, as individuals, are impressed by a machine’s ability that is predicated on near-instantaneous access to a memory of ever-expanding capacity. What looks like applied reasoning is, in fact, no more than “regurgitating information they’ve already been trained on.” He adds, “You can manipulate language and not be smart, and that’s basically what LLMs are demonstrating.”
His trenchant views will not go down well with the majority of established and startup AI companies that reckon large language models will be the easiest and quickest route to AGI and are betting the farm on that belief.
Multimodel AI: A shiny new tool but basically still a blunt instrument
That’s not to say that the developments of multimodal AI will not be impressive and useful. For example, the latest natural language processing (NLP) technologies can already provide remarkable speech recognition and speech-to-text capabilities, together with speech or text-to-speech outputs and translations between the world’s languages. The most modern NLP developments can even recognise vocal tics associated with sarcasm, irony and stress, as well as local dialects, idioms and slang.
Elsewhere, advances in computer vision have now progressed well beyond the ability simply to identify objects. The amalgamation of multiple data types helps multimodal AI to identify and specify the context of an image and make more accurate determinations based on those observations. The image of a duck swimming in a pond, when combined with the sound of one quacking, is much more likely than not to result in the accurate identification of the object as a duck.
Thus, in this circumstance, multimodal GenAI might be seen to be able to perform the abductive reasoning that “if it looks like a duck, swims like a duck, and quacks like a duck, then it probably is a duck”. Abductive reasoning is based on making an inference, from observations, about which of several explanations for a particular event or thing is the most likely. Humans can very quickly identify an unknown subject by observing its habitual characteristics, but so can multimodal GenAI. The question is, does the AI reach its answer in the same way as a human? The philosophers are cogitating and the jury’s out.
Multimodal AI is particularly pertinent in robotics and its interaction with human environments: They apply data from cameras, microphones, GPS and many other sensors to create a detailed understanding of where they are and what they are doing. However, all multimodel AI is, in the end, limited by the sheer volume of the data required for them to operate, its quality and how and where that data is stored. Storing ever-increasing volumes of data in massive datacentres is very expensive, as is processing it.
What’s more, humans are finding it difficult to understand how the neural networks that multimodal AI develop actually work and how data is appraised and decisions autonomously made. That is making it difficult to determine in-built bias in the AI decision-making process and even more difficult to fix bugs and eliminate mistakes especially when new data is added to its training set. The concern is that multimodal AI will be both unpredictable in its operation and unreliable in its decision-making and, for the present at least, that makes multimodal AI as a tool to help humanity more of a sledgehammer than a scalpel.
– Martyn Warwick, Editor in Chief, TelecomTV
Email Newsletters
Sign up to receive TelecomTV's top news and videos, plus exclusive subscriber-only content direct to your inbox.